Class is moving fast.
- The basics quickly gave us the language to write and speak about small data sets often in a normal distribution often with a little bit right skewed. Lots of histograms.
- Central Limit Theorem: In probability theory, the central limit theorem establishes that, in some situations, when independent random variables are added, their properly normalized sum tends toward a normal distribution even if the original variables themselves are not normally distributed. (Wikipedia)
- Sample data sets have let led us through Python exercises studying where the mean of the data is. Lots of contingency tables.
- We created a training and test data set. The ratio of the random sample split was about 5 to 1.
- Probability and set theory. Which brings us to the featured topic.
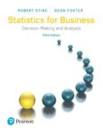
Statistics for Business:
Decision Making and Analysis,
3rd. Edition.
The Sharpe Ratio
"Which random variable represents the better investment going forward?
Finance offers many ways to judge the relative size of rewards in comparison to risks. One of the most popular is the Sharpe ratio. The higher the Sharpe ratio, the better the investment.
The Sharpe ratio is a fraction derived from percentage changes in the value of a stock. The numerator of the Sharpe ratio is the mean return minus what you would earn on an investment that presents no risk. The denominator of the Sharpe ratio is the standard deviation of the return on the investment. Suppose X is a random variable that measures the performance of an investment, and assume that the mean of X is μ and its SD is σ.
The Sharpe ratio of X is ratio of an investment’s net expected gain to its standard deviation."
Comments